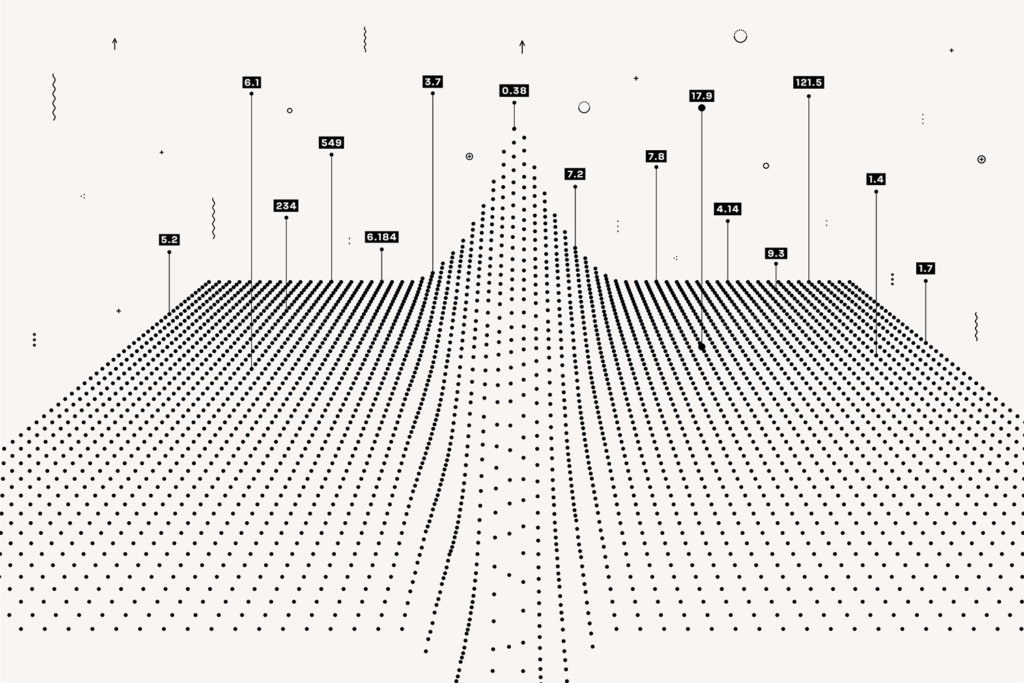
How to create a consistent data experience across your data ecosystems
Companies are increasingly migrating from fragmented, monolithic data platforms to more streamlined and flexible data ecosystems. This is in order to respond to the changing market and provide tailored customer services, as well as a more consistent digital experience. And, in times of unexpected crises like COVID-19, a unified and flexible data ecosystem is critical. Chandra Devarkonda of EY tells us more.
New data ecosystems, such as those offered by public cloud providers, are attractive migration options. However, instead of moving all of their data to a single public cloud provider, many companies are mitigating risk by diversifying across several providers, as well as retaining some critical data within their internal private data ecosystems. This hybrid approach opens up several new opportunities for business agility, but also presents new challenges in data management.
Customer trust, system capacity and managing costs are critical to survival in times of unexpected crises, like the one the world is currently going through. Fortunately, these challenges can be resolved through a more modern architecture approach called the ‘data fabric’, or data plane, that provides a unified, elastic method to data management across any number of ecosystems.
What is data fabric?
Data fabric is a set of independent services that are stitched together to provide a single view of your data, irrespective of the repositories where it is generated, migrated to or consumed from. These services, built using artificial intelligence (AI) methods and modern software engineering principles, include business services, data management services, monitoring services, a data catalogue and more. Services can also track data and attribute value to it through its lifecycle, thereby informing data resiliency and lifecycle management planning while also providing the flexibility to apply tiered data quality, privacy and security solutions.
As a result, organisations will be better able to evolve alongside changes in business needs as well as technology. This approach is designed to be long-lasting and forward-looking, and can cater to a variety of upcoming technology trends.
What you gain through data fabric
A data fabric approach to provisioning and managing large-scale networks of services that abstract—or reduce to essentials for the end user—business, data and infrastructure functions across hybrid and multi-cloud data ecosystems enables organisations to reliably manage data, while simplifying the implementation of consistent policies across these ecosystems.
In this digitally disruptive age, automatic abstraction of commonly used business functions, centralising key data management functions and better managing infrastructure for storage, computation and access will help organisations increase focus, mitigate infrastructure costs and better allocate resources for creating business value. Let’s explore these benefits in greater detail.
Abstraction
Each of the independently hosted services that comprise the data fabric help organisations abstract the functions the services provide. While the services are primarily targeted at the chief data officer (CDO) and chief information officer (CIO) levels to manage data and infrastructure, they also impact functions such as marketing, customer service and finance.
Consider the marketing function. If marketers want to more nimbly test new offers or services, such as by comparing the effectiveness of different web pages or product offers by customer segment and rolling out continuous updates, they can use specific services hosted on the data fabric that encapsulate all the necessary functions and provide that visibility.
Likewise, in finance functions, independent financial calculation functions such as loan amortisations or cash flow calculations are hosted on the data fabric and updated without disrupting how they are accessed. Data usage by these functions is also independent of where such data is stored. Data quality checks are automatically built so the trust in usage of the data significantly increases.
Trust and risk mitigation
Improved lineage, traceability, explainability and transparency form the core of future-focussed governance.
By consistently applying identification, tagging and controls to your data elements, along with privacy screening as needed, you mitigate risk. And consistent data experiences, privacy applications and data quality improve trust between the organisation and its customers.
To gain insight into the type of privacy screen to be applied when data is consumed, an organisation can tag sensitive data non-intrusively, track and assign qualitative metrics based on cost of acquisition, and process and store consumer data using operational logs and business rules. The privacy group of services can enable such functionality while also using AI to apply configurable privacy screens. They also enable use of natural language processing to digitise new privacy rules and incorporate them into the screening services without much human intervention needed.
Economic modelling
At the infrastructure level, an economic utility service on the data fabric determines data storage and computer needs through a cost-benefit analysis, for when multiple data centres are being managed. The economic model uses workload costs and several historical analyses of similar workloads to help make a more informed decision on the type of infrastructure needed to execute the tasks across public cloud ecosystems. Additionally, an organisation can better match and distribute workloads across data centres through simulations.
Data fabric architects and engineers can design and implement the specific services that will help you manage diverse data ecosystems across several cloud platforms. Consultants may use a pilot prototype for demonstration and as part of a workshop to assess your needs and evaluate where you need help.
Data fabric in action
To illustrate the challenges, we’ll use a case from a global insurance organisation that is considering using a product-pricing application across its internal data centre and on a public cloud.
The application needs to scan through terabytes of data (not all of which is available on the public cloud), select valuable data points as inputs and then execute a complex set of mathematical functions iteratively to arrive at a possible price.
This price needs to be accessible by a customer through the organisation’s website and mobile app. It also needs to be recorded internally.
The organisation carries out the pricing calculations on the public cloud, where it is far easier to spin up the hardware and software that match the needs of the pricing application, as well as to access the data, perform the calculations and generate the prices. However, the organisation also needs to continue using its internal data systems due to dependent applications and incumbent business processes.
This poses several different challenges, including:
- How does the organisation know beforehand how much infrastructure it would need to run the pricing calculations?
- What if the organisation has some idle capacity in its internal data systems that could be used along with the additional public cloud capacity?
- If the organisation uses multiple cloud vendors, how can it decide quickly how to federate the application execution to optimise the overall costs?
- How can the metadata being captured in internal data systems be linked on a continuous basis with that on the cloud to provide a consistent experience?
- If the application needs to provide a price to millions of customers worldwide in real-time, how can one be sure it is providing an accurate price that is calculated at the speed demanded, as well as transparency on how it arrived at that price in a consistent manner?
- How can new business rules be injected into the pricing application that incorporate changing market factors as well as local market regulations in a globally consistent manner?
This multitude of different challenges is addressable by using the menu of services offered and hosted on the data fabric. Each service will address a specific data challenge, and is itself composed of several sub-services, each performing an independent function. For example: one sub-service would capture relevant data; the second perform validation checks; the third apply advanced algorithms to learn about the data; and the fourth could offer a recommendation or provide an alert. Each of these sub-services can be managed and updated without disrupting the overall service. The data fabric services, while independently functioning, are meshed together through the public cloud platforms and hosted in a cloud-based data centre of the organisation, removing the need to manage infrastructure to host the services.
This makes data management more automated, anonymous and scalable across ecosystems. It also allows organisations to manage data across myriad platforms though a unified layer, greatly abstracting from their data infrastructure while also providing transparency.
Disclaimer
This material has been prepared for general informational purposes only and is not intended to be relied upon as accounting, tax or other professional advice. Please refer to your advisors for specific advice..
EY is a global leader in assurance, tax, transaction and advisory services. The insights and quality services we deliver help build trust and confidence in the capital markets and in economies the world over. We develop outstanding leaders who team up to deliver on our promises to all of our stakeholders. In so doing, we play a critical role in building a better working world for our people, for our clients and for our communities.
Recent Comments